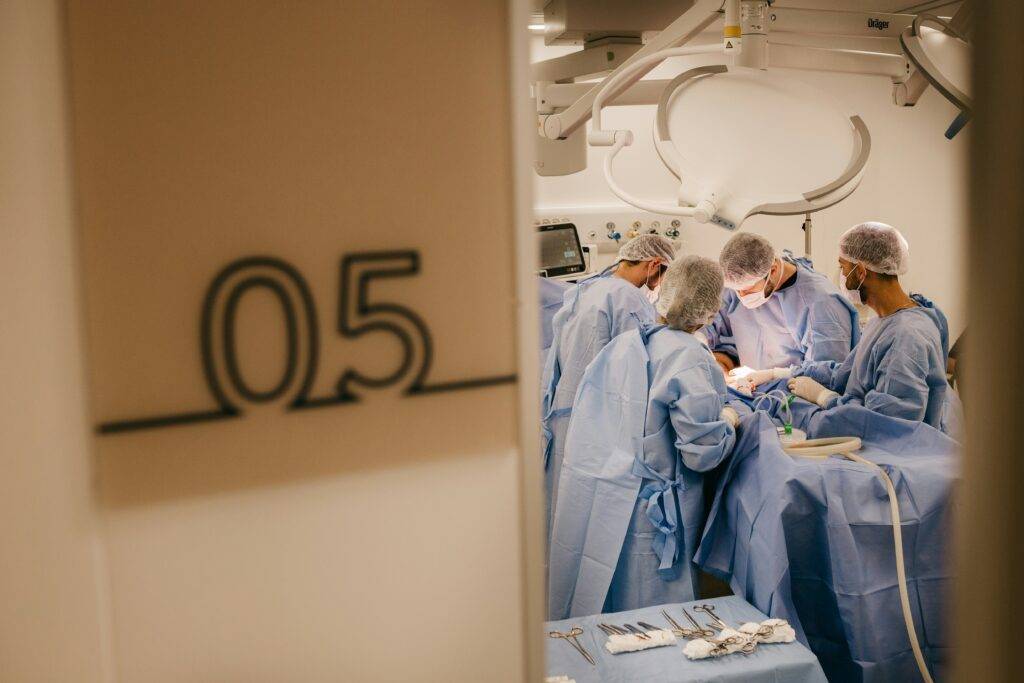
Generative AI and Clinical Efficiency
Artificial Intelligence (AI) has acquired a competitive and radical character across numerous fields and healthcare is among the hardest hit. However, within the healthcare sector, generative AI, which is AI that is able to produce content in many forms like text, images, and also recommends action, has come in handy in increasing the clinical efficiency. It is dealing with many long self-inflicted problems within the health care, such as clinician burnout, administrative work load, poor patient clinical outcomes, and many others, through processes automation, utilizing soil accurately, and also customizing care to the patients.
In the following article, we would look into what generative AI really means, how it has made it possible to improve clinical outcomes, and how it would be used in the coming years and its challenges.
Defining Generative AI(Artificial Intelligence) within the Context of Healthcare
Generative AI is an umbrella term for different models of AI that generates new data based on old data they have been transforming/ trained by. For example, in the health care field, this can range anywhere from generating narratives (such as medical notes) to generating synthetic imaging data towards generating a more personalized treatment outcome for the patient’s medical documentation history. Recently many advanced Algorithms like the Large Lantern Models are developed to receive training in clinics with genuine patients and practitioners order.
Approaches to Occupational Generative AI(Artificial Intelligence) Technology.
The basis of generative AI(Artificial Intelligence) in healthcare is its ability to:
Issues related to data volume in healthcare can be successfully handled and managed by AI in real-time which goes far beyond, human intelligence.
Create bridging innovative recommendations that do not exist within existing data by using patterns found.
Adapt along with the advancing knowledge of medicine to ensure that the recommendations provide to the clinicians are up-to-date and supported by the most recent evidence
Generative AI in Administrative Efficiency
One of the areas that has seen an immediate impact of generative AI in healthcare is in enhancing the efficiencies in eliminating administrative workload. Studies have shown that a significant part of the clinician’s working hours actually goes to non-clinical duties, such as form filling, meticulating patients information and dealing with red tape. This cuts the interaction time with the patients and adds to the reasons for clinician burnout.
Generative AI can enhance these administrative responsibilities in the following ways:
AI Scribes: Clerical duties have been carried out by AI in the last couple of decades, especially during patient interaction when there is a need to produce detailed notes about the encounter. Clinical visits with all their overheads promote increase levels of healthy patient care. AI scribes have been shown already to make clinicians’ documentation less tiresome and more productive who will then be more devoted to patient treatment.
EHR Optimization: Although Electronic Health Records (EHRs) are indispensable, they are frequently too difficult to work with. Patient records can be summarized generative AI tools, assistance at data entry can be provided and any relevant information can pulled together in such a way that the practitioners have an overall picture of the patients’ health in less time. Sometimes referred to as keyboard liberation, this process is meant to help clinicians work less with the computer and more with the patients. At least this is what they say.
Automation of Routine Tasks: Parts of a processes which are circumstantial but normal like appointment, taking patient’s details, and dealing with the payer systems are automated especially with generative AI tools. These systems are capable of eliminating tasks that staff would have taken a longer time to accomplish and with more mistakes creating shorter time frames in treating patients and low operation costs.
How Better Clinical Decision-Making is Achievable in the Future
Real time analyzing of the patient information is done by the Generative AI models and subsequently directs these models in what the clinician should do. The ai can be quite useful in complex cases where the clinician is not able to see quite a lot of patterns or potential diagnoses at that moment.
Predictive Analysis and Risk Assessment: Medical Generative AI systems can examine a patient’s health history, genetic records, and medical conditions to provide a prognosis of possible future health events. For instance, AI can help screen individuals more prone to diabetes or heart disease and offer timely intervention measures to avert these conditions.
Support in Diagnosis: There are well-documented instances where AI has been helpful when it comes to diagnosis, notably, in the field of radiology where it can process medical imaging data far quicker and often more accurately compared to human operators. MORE, Generative AI systems could assist radiologists by automatically flagging radiographs where there is a possibility of disease, freeing them up to assess the more complicated cases. It has been demonstrated in some projects involving AI-aided diagnosis that the patients’ error rates and clinical outcomes can be improved.
Diagnostic Prediction: The new trends in artificial intelligence enable people to pursue development of treatment recommendation systems which rely not only on medical literature but rather on available clinical guidelines and patient characteristics. This makes sure that the patients are embarked into a regimen which is both customized with respect to the patient but also conformed to the most current clinical research.
Improving Communication With Patients
The aspect of generative artificial intelligence that needs to be highlighted in this paper is its usefulness in improving the quality of interactions between patients and clinicians. Virtual health assistants which embrace AI technologies have started advising patients on how they should proceed in regards to their consultation including welcoming patients and gathering health information or recommending health options.
Healthcare Coaching: There is capacity for AI models such as LLMs to act as healthcare coaches for patients helping them in controlling diseases to which patients are prone into forget medication, assess symptoms and suggest appropriate modifications to lifestyle. These virtual coaches are accessible at any time of the day throughout the week without restrictions and are capable of providing an additional level of continuous support which compliments the more conventional face to face care of the patients.
Patients and Their Feedback: Generating these consultations can help to accept innovations, including interactive consultation with patients for data collection Instead of this Korean Survey giving static forms to patients, AI systems could be used that will ask to fill in the necessary information and provide feedback guiding patients through questions. This ensures better quality data is collected, and even the errors in self reporting by patients are minimized.
Generative AI in Research and Drug Development.
The recent advancement of an AI in terms of data management is also able to disrupt the scope of drug discovery and the conduction of clinical trials. The entire conventional drug development cycles are relatively time consuming and costly, however, the use of generative AI, through modeling biological systems, forecasting the behavior of specific molecules and rational drug design, could shorten them.
AI-Driven Drug Discovery: AI-led drug discovery remains the fastest in discovering new drug targets through screening hundreds and millions of databases at molecular, cell, and tissue levels than any other traditional method. The application of Generative AI models is that they are trained to spot novel relationships among data that have become too complex for human scientists and therefore assist in the formulation of drugs for complicated diseases such as cancer and Alzheimer’s (Light-it Health).
Optimizing Clinical Trials: Clinical trials are crucial in the process of testing any new medication, yet it is most common to face issues that hinder patient recruitment and data collection from them during clinical trials. AI would step in to aid forecast and identify the patients’ most suitable and likely to respond to the trial, thus optimizing the trial and enhancing the chances of success. Additionally, with the help of generative AI, researchers may create more efficient trial procedures and forecast outcomes based on what data has been collected in the past.

Understanding Health Workforce Problems
Like all industries, the healthcare sector is also beginning to suffer from a shortage of willing workers and by 2030, the World Health Organization projects a deficit of over 10 Million healthcare workers. Generative AI is seen as a possible cure for this problem.
Extending the Workforce with AI: Healthcare staff should not just be increased but enhanced. AI can handle many of the time-consuming activities and lighten some parts of the care of the patients allowing the healthcare professionals to shift their attention towards more demanding portions of the care. This could contribute to reducing the strain on the already stressed personnel and thus, enhance the provision of health services in general.
Artificial Intelligence Assistance in Nursing: AI virtual assistants can help remotely care for the patient by checking vital signs, responding to queries, and advising patients, so nurses will be able to look after more patients. There are especially useful assistants in the management of chronic diseases where patient education and adherence can spell the difference between poor and good outcomes.
Hurdles and Implications
While the positives of generative AI in the healthcare sphere are rather apparent, there are hurdles and moral issues that are still shunned.
Data Security: This problem is twofold. Effective generative AI systems can be, able to operate in confined spaces because they can process massive quantity of patient information. Hence, patient data privacy and protection is of utmost importance, particularly given certain regulations such as the general data protection regulation in the EU and the health information portability and accountability act in the US. Bias in AI Models: AI Models Are Only As Good As The Data With Which Noisemaincommead.
In the event that there are any biases in the training data or a lack of diversity, the machine learning algorithm will be able to produce biased results resulting in inequality and therefore a disparity in care provision. It is essential that AI models are built on wide datasets training in order to promote lack of rationality and this cases regular monitoring of the systems by qualified personnel on the other hand.
Job Displacement: While there is, AI takes over more and more functions, there are worries about unemployment, especially in administrative and less paid health sector work. As AI technology can reduce the volume of work, it is very possible that the number of these empty positions can seldom apply more raising upon how the transition of the current labor force is to be managed.
Conclusion: The Power of Generative AI(Artificial Intelligence) in Healthcare Industry
In their most effective generation, help, and of course, creativity, Generative AI(Artificial Intelligence) has strong impulses and capabilities, particularly in the improvement of clinical practice, cost control, and better overall patient care. Automating monotonous activities, assisting in clinical decision making, and delivering specific patient interventions, AI addresses some of the problems recognized within the current healthcare systems: shortage of workforce, growing demand, and cost containment.
Nevertheless, these opportunities could only be realized if organizations of care industries comprehend and deal with the ethical and practical limitations of artificial intelligence such as patient data, discrimination, and where the future of health workers lies. If appropriate governance structures and mechanisms are adhered to, Generative AI will be relied on as a tool for enhancing the delivery of health services and improving the health of the population. In this special time, all things are hampered, except that improvement in healthcare practice would easily achieve.
13 thoughts on “What do you need to know Generative AI(Artificial Intelligence) and Clinical Efficiency: Changes Initiated In Modern Healthcare”