Introduction
AI in the Healthcare There are numerous ways in which Artificial Intelligence (AI) can disrupt and advance the healthcare system by improving patient care outcomes, enhancing medical ways of working efficiently and providing health care cheaper and all-inclusive. AI technology is harnessed with a range of applications like the machine learning techniques, predictive analytics, and natural language processing to help in disease diagnosis,mode of treatment, and storage of medical information.
While the promises of AI in health care are enormous and weighty, the embrace of these systems into everyday clinical practice is fraught with notable issues. System failures include an ethical paradigm, data security threats, and privacy enabling fabrications of the medical prospective. This article analyzes the opportunities presented by AI in the course of delivering health services while addressing the threats of system failure with regard to ethical behaviour, computer security, and data accuracy. AI in the Healthcare
The Gains from the Introduction of AI in the Healthcare Industry
The application of AI more importantly to health care has much entered the phase that will erect and expand on the current health care industry as well as create simply new industries emphasizing on value provision. AI in the Healthcare
Enhanced Diagnosis and Early Detection:
AI-based diagnostic tools are capable of deciphering complex medical images like the X-Ray, MRI, and CT scan with high levels of accuracy. The machine learning algorithms will be able to analyze these images and study parts that may be overlooked by medical professionals, which leads to the treatment of diseases such as cancer, heart problems, and Kim 13 neurological disorders at an early stage. AI can improve the efficiency of diagnosis, thus cutting down the waiting time for treatment.
Customized Treatment Plans:
Applying AI systems to huge amounts of data about each patient, genetic, environment, and even lifestyle enables the creation of personalized treatment approaches. With the above strategy, which is termed as precision medicine, medical practitioners administer the most suitable interventions based on the patient’s disease profile, improving the patient’s condition and reducing the side effects. AI in the Healthcare
Operational Efficiency:
AI systems can also assist with administrative duties such as patient appointment scheduling, patient billing, and maintaining patient records, hence relieving the health worker from non-patient care responsibilities. Hospitals can use predictive analytics to predict patient admissions, appropriate staffing, and cut down waiting time in order to achieve effective resource management.
According to specialists in drug design processes, development of drugs is greatly helped by the implementation of artificial intelligence into the knowledge based technologies. For instance, by taking into consideration a large set of chemical compounds, the expectation is that AI can determine which compounds will work towards curing which diseases. Such an outcome could lead to reduced time and costs incurred in devising treatments, which in turn may hasten the arrival of cures to the market. AI in the Healthcare
AI in the Healthcare System Flaws and Risks
The AIs possibilities in the field of healthcare are numerous, however, the concern issues attendant to the introduction of AI systems can be quite worrying, related as they are to issues of ethics, safety, and data accuracy. These risks are threat to health and well being of patients, or protection of their personal data and trust in the health system.
AI in the Healthcare Ethical Challenges
AI in the Healthcare There are several ethical challenges that accompany the use of AI that are hard to mitigate, with regard to decision making, responsibility and equity.
Bias and Discrimination: AIs are designed to learn from past activity by utilizing past activity. Hence, they destroy diversity regardless of whether AI training exempts it. A single example is illustrative: An AI that is fed with medical images that have been collected mostly from masculine populations will be biased towards providing diagnosis and treatment for males. This has very serious implications for health equity and health justice.
Opacity: The decision-making architecture of many AI systems, particularly those featuring deep structures, corresponds to a black box in which the inner workings are not easily amenable to human comprehension and this poses a challenge especially when implementing AI in health care, Levin, 2020. Many questions of ethics arise from the very same issues – who is actually assuming the responsibility? — the AI system, the physician, the organization? In this layer of engagement patients do not act but interact with computer systems that run on the AI.
Patient Autonomy: Thus, it is not unusual for AI systems to accumulate vast amounts of patient information. We wish to raise concerns regarding whether patients have a clear understanding of the data used and the over that they have provided consent. Therefore the traditional relationship between a patient and a doctor where the patient plays an integrative activity in their treatment is likely to change due to the advancement in AI systems.
Security and Data Privacy Risks
AI in the Healthcare AI application in health care retains the need for the integration of patient health records that comprise sensitive information and therefore data loss prevention is an issue that warrants attention.
Data Breaches and Cybersecurity: Specialty clinics and hospitals are very prone to cyberattacks due to a huge amount of data, a risk which is also directed against the artificial intelligence systems in charge of huge data sets. Cases of breaches of patients data span from reputation abuse, financial breach, identity inclusion theft and many more consequences to the concerned healthcare agencies. The use of AI systems in the manner, whereby they straddle different platforms and devices, creates more avenues through which cybercriminals can launch attacks and hence security is more difficult to manage.
Data Ownership and Control: This is specifically apparent in the case of AI systems which are used over the cloud or where such systems adopt the use of third party providers. Who owns the patient data that is being processed by AI systems? The Medical professional, the AI enterprise, or the individual patient? Issues that arise out of the lack of this clarity are legal tussles and issues regarding controversy on how data is handled, who it is shared with and how it is protected.
Inadequate Privacy Protections: Issues of healthcare related AI systems and design may present an unintended feature that exposes patient information from leakage. Models built from real world patient information data may pose the risk of personal information, medical or otherwise types of breaches thus endangering patient relations ‘satisfaction’ and the AI can prove useful for.
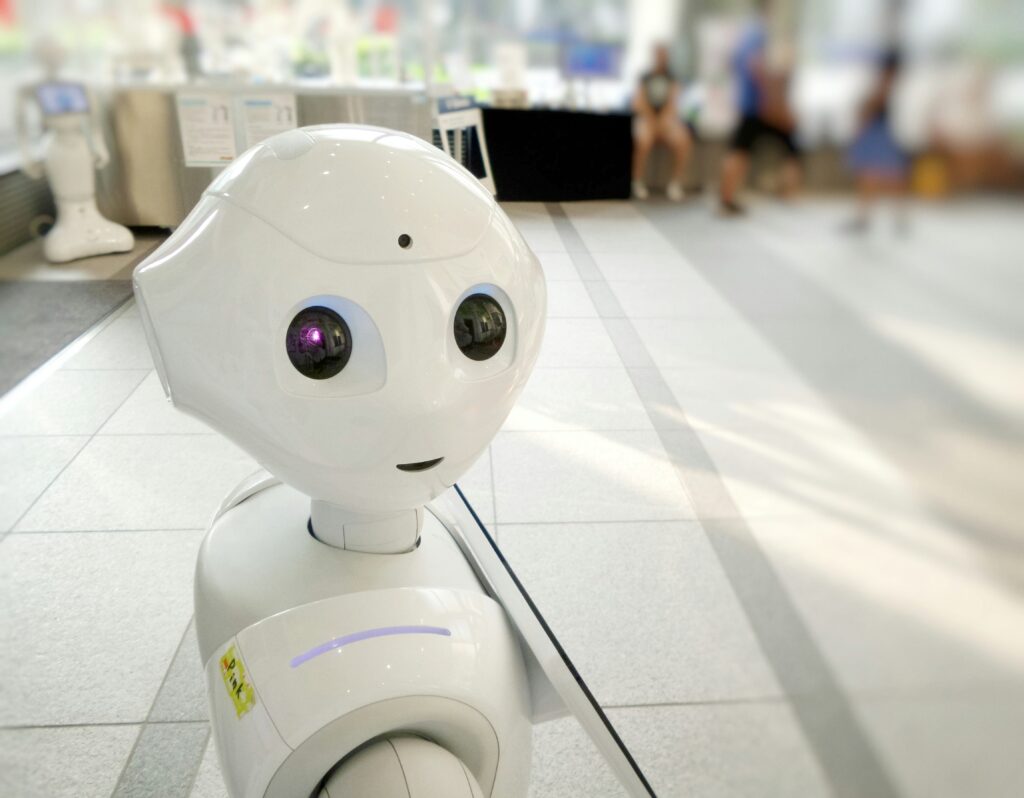
Risks to Data Accuracy
Sufficient and reliable data is a basic necessity and ideal requirement to successfully deploy and utilize effective AI multi-level platforms in healthcare however some factors compromise data quality and data accuracy, which in turn affect patient care.
Inaccurate or Incomplete Data:
What makes AI less effective than its human instructors? It’s inaccurate or incomplete data. It is very clear that any misleading or missing information will result in predicting or diagnosing the problem wrongly. For instance, if the available patient records in the system are not maintained by regularly making updates, the AI systems might recommend errors based on old information.
Data Fragmentation:
Healthcare data, like any other data, is often kept in a lot of different basements that do not speak to on another, at the same time making individual issues become registers. This is especially the case with AI systems. Using partial data to draw conclusions puts these systems at risk of losing out on very important facts, leading to the issuing of useless tips or even harmful ones.
Algorithmic Errors:
All AI systems can most of the times be put into error despite them being well structured. The machine learning model may face difficulty in making inferences from the training data set when dealing with real cases, especially when new or strange cases are presented. These algorithmic biases can be the basis of placing wrong diagnosis on patients or planning various treatment strategies that should not be used for the patients which puts their lives at risk.
Balancing AI’s Potential with Responsible Implementation:
To the maximum extent possible, the AI in medicine must be efficient while systematic flaws cannot be effectively eliminated unless they are noted and therefore a well-structured process is followed throughout the implementation of the system.
Ethical AI Design:
All designs of the AI systems should be carefully represented in fairness, accountability, and transparency. This involves assessing datasets for bias, designing models that can be interpreted by a layperson where possible, and creating environments where providers and patients can question and explain the use of AIs and their findings.
Data Security and Privacy Protections:
Patients and their biological data should be protected by formidable security measures that may include encryption, two-step verification, and frequent testing of the system. There is no proper mechanism in place to ensure the protection of data ownership, consent on the dat, and policies that prevent everybody from abusing patients over their own health data.
Improving Data Quality:
There should be an assurance that all the needed information necessary for the use of AI systems in clinical practice, is available, relevant, and current. There is going to be a need to unify EHR systems and enhance the integration of various healthcare IT systems, in order to eliminate information silos and enhance the accuracy of machine intelligence.
Regulatory Oversight:
Regulatory agencies and the government must develop regulations concerning the implementation of AI systems in the healthcare sector. This also involves the establishment of acceptable standards for data protection and privacy, ethical issues, and more importantly, high-level quality assurance of the implemented AI systems before being used in actual practice.
Conclusion
Healthcare, as we know it, is destined to be reincarnated with the adoption of artificial intelligence in it, with enhanced diagnosis, tailor-made therapies and optimized work processes. Nonetheless there are issues concerning the system reliability regarding moral, safety and data quality perspectives that need understanding. As such, and with the aid of responsible evolution, strong safety measures and moral supervision, the health system will fully able to pick all the benefits of AI while protecting patients and their privacy. Unequivocally, the answer lies in finding an equilibrium where the embracement of novel ideas does not compromise the application of emerging technologies in the healthcare system.